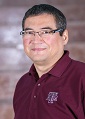
Erchin Serpedin
Texas A&M University, USA
Title: A robust PCA algorithm for metagenomic biomarker detection
Biography
Biography: Erchin Serpedin
Abstract
We propose a novel consistency-classiï¬cation framework that enables the assessment of consistency and classiï¬cation performance of a biomarker discovery algorithm. The proposed evaluation protocol is based on random resampling those models the variation in the experiment size. The metagenomic data matrix is modeled as a superposition of two matrices. The ï¬rst matrix is a low-rank matrix that depicts the abundance levels of the irrelevant bacteria. The second matrix is a sparse matrix that describes the abundance levels of the bacteria that are differentially abundant between different phenotypes. We propose a novel Robust Principal Component Analysis (RPCA) based biomarker discovery algorithm to recover the sparse matrix. RPCA is a multivariate feature selection approach that processes the features collectively rather than individually. Comprehensive comparisons of RPCA with the state-of-the-art algorithms on two realistic datasets show that RPCA consistently outperforms the existing state-of-the-art algorithms in terms of classiï¬cation accuracy and reproducibility performance. Thus, the proposed RPCA-based biomarker detection algorithm provides a high reproducibility performance irrespective of the complexity of the dataset and the number of selected biomarkers. RPCA selects also biomarkers with quite high discriminative accuracy. Therefore, RPCA appears to represent a very consistent and accurate methodology for selecting taxonomical biomarkers in microbial populations.